計量經(jīng)濟學(xué)英
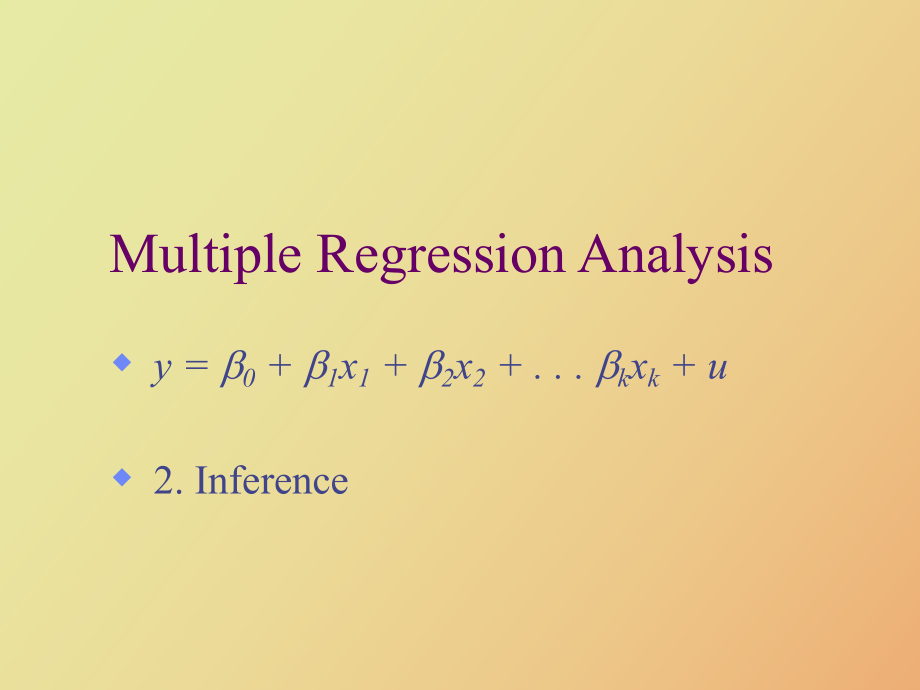


《計量經(jīng)濟學(xué)英》由會員分享,可在線閱讀,更多相關(guān)《計量經(jīng)濟學(xué)英(34頁珍藏版)》請在裝配圖網(wǎng)上搜索。
1、Multiple Regression Analysisw y = b0 + b1x1 + b2x2 + . . . bkxk + uw 2. Inference Assumptions of the Classical Linear Model (CLM) So far, we know that given the Gauss-Markov assumptions, OLS is BLUE, In order to do classical hypothesis testing, we need to add another assumption (beyond the Gauss-Mar
2、kov assumptions) Assume that u is independent of x1, x2, xk and u is normally distributed with zero mean and variance s 2: u Normal(0,s2) CLM Assumptions (cont) Under CLM, OLS is not only BLUE, but is the minimum variance unbiased estimator We can summarize the population assumptions of CLM as follo
3、ws y|x Normal(b0 + b1x1 + bkxk, s2) While for now we just assume normality, clear that sometimes not the case Large samples will let us drop normality .x1 x2The homoskedastic normal distribution with a single explanatory variable E(y|x) = b0 + b1xyf(y|x) Normaldistributions Normal Sampling Distribut
4、ions errors theofn combinatiolinear a is it becausenormally ddistribute is 0,1Normal thatso ,Normal st variableindependen theof valuessample the on lconditiona s,assumption CLM Under thejb bbb bbb jjj jjj sd Var The t Test 1:freedom of degrees theNote by estimate tohave webecause normal) (vson distr
5、ibuti a is thisNote sassumption CLM Under the 221j knt tse knjj ssbbb The t Test (cont) Knowing the sampling distribution for the standardized estimator allows us to carry out hypothesis tests Start with a null hypothesis For example, H0: bj=0 If accept null, then accept that xj has no effect on y,
6、controlling for other xs The t Test (cont) 0j H ,hypothesis null accept the o whether tdetermine torulerejection a withalong statistic our use then willWe : for statistic the form toneedfirst eour test w perform To t sett jjj bbb b t Test: One-Sided Alternatives Besides our null, H0, we need an alte
7、rnative hypothesis, H1, and a significance level H1 may be one-sided, or two-sided H1: bj 0 and H1: bj 0c0 a1 aOne-Sided Alternatives (cont)Fail to reject reject One-sided vs Two-sided Because the t distribution is symmetric, testing H1: bj 0 is straightforward. The critical value is just the negati
8、ve of before We can reject the null if the t statistic than c then we fail to reject the null For a two-sided test, we set the critical value based on a/2 and reject H1: bj 0 if the absolute value of the t statistic c yi = b0 + b1Xi1 + + bkXik + uiH0: bj = 0 H1: bj 0c0 a/21 a-ca/2Two-Sided Alternati
9、vesreject rejectfail to reject Summary for H0: bj = 0 Unless otherwise stated, the alternative is assumed to be two-sided If we reject the null, we typically say “xj is statistically significant at the a % level” If we fail to reject the null, we typically say “xj is statistically insignificant at t
10、he a % level” Testing other hypothesesA more general form of the t statistic recognizes that we may want to test something like H0: bj = aj In this case, the appropriate t statistic is teststandard for the 0 where, j jjja seat bb Confidence Intervals Another way to use classical statistical testing
11、is to construct a confidence interval using the same critical value as was used for a two-sided test A (1 - a) % confidence interval is defined as ondistributi ain percentile 2-1 theis c where, 1 kn jj t sec abb Computing p-values for t tests An alternative to the classical approach is to ask, “what
12、 is the smallest significance level at which the null would be rejected?” So, compute the t statistic, and then look up what percentile it is in the appropriate t distribution this is the p-value p-value is the probability we would observe the t statistic we did, if the null were true Stata and p-va
13、lues, t tests, etc. Most computer packages will compute the p-value for you, assuming a two-sided test If you really want a one-sided alternative, just divide the two-sided p-value by 2 Stata provides the t statistic, p-value, and 95% confidence interval for H0: bj = 0 for you, in columns labeled “t
14、”, “P |t|” and “95% Conf. Interval”, respectively Testing a Linear Combination Suppose instead of testing whether b1 is equal to a constant, you want to test if it is equal to another parameter, that is H0 : b1 = b2 Use same basic procedure for forming a t statistic 21 21 bb bb set Testing Linear Co
15、mbo (cont) 2112 2112222121 212121 2121 , of estimatean is where 2 ,2 then,Since bbbbbb bbbbbb bbbb Covs ssesese CovVarVarVar Varse Testing a Linear Combo (cont) So, to use formula, need s12, which standard output does not have Many packages will have an option to get it, or will just perform the tes
16、t for you In Stata, after reg y x1 x2 xk you would type test x1 = x2 to get a p-value for the test More generally, you can always restate the problem to get the test you want Example: Suppose you are interested in the effect of campaign expenditures on outcomes Model is voteA = b0 + b1log(expendA) +
17、 b2log(expendB) + b3prtystrA + u H0: b1 = - b2, or H0: q1 = b1 + b2 = 0 b1 = q1 b2, so substitute in and rearrange voteA = b0 + q1log(expendA) + b 2log(expendB - expendA) + b3prtystrA + u Example (cont): This is the same model as originally, but now you get a standard error for b1 b2 = q1 directly f
18、rom the basic regression Any linear combination of parameters could be tested in a similar manner Other examples of hypotheses about a single linear combination of parameters: n b1 = 1 + b2 ; b1 = 5b2 ; b1 = -1/2b2 ; etc Multiple Linear Restrictions Everything weve done so far has involved testing a
19、 single linear restriction, (e.g. b1 = 0 or b1 = b2 ) However, we may want to jointly test multiple hypotheses about our parameters A typical example is testing “exclusion restrictions” we want to know if a group of parameters are all equal to zero Testing Exclusion Restrictions Now the null hypothe
20、sis might be something like H0: bk-q+1 = 0, . , bk = 0 The alternative is just H1: H0 is not true Cant just check each t statistic separately, because we want to know if the q parameters are jointly significant at a given level it is possible for none to be individually significant at that level Exc
21、lusion Restrictions (cont) To do the test we need to estimate the “restricted model” without xk-q+1, , xk included, as well as the “unrestricted model” with all xs included Intuitively, we want to know if the change in SSR is big enough to warrant inclusion of xk-q+1, , xk edunrestrict isur and rest
22、ricted isr where,1 knSSR qSSRSSRF ur urr The F statistic The F statistic is always positive, since the SSR from the restricted model cant be less than the SSR from the unrestricted Essentially the F statistic is measuring the relative increase in SSR when moving from the unrestricted to restricted m
23、odel q = number of restrictions, or dfr dfur n k 1 = dfur The F statistic (cont) To decide if the increase in SSR when we move to a restricted model is “big enough” to reject the exclusions, we need to know about the sampling distribution of our F stat Not surprisingly, F Fq,n-k-1, where q is referr
24、ed to as the numerator degrees of freedom and n k 1 as the denominator degrees of freedom 0 c a1 af(F) FThe F statistic (cont)rejectfail to reject Reject H0 at a significance level if F c The R2 form of the F statistic Because the SSRs may be large and unwieldy, an alternative form of the formula is
25、 useful We use the fact that SSR = SST(1 R2) for any regression, so can substitute in for SSRu and SSRur edunrestrict isur and restricted isr again where,11 2 22 knR qRRF ur rur Overall Significance A special case of exclusion restrictions is to test H0: b1 = b2 = bk = 0 Since the R2 from a model wi
26、th only an intercept will be zero, the F statistic is simply 11 2 2 knR kRF General Linear Restrictions The basic form of the F statistic will work for any set of linear restrictions First estimate the unrestricted model and then estimate the restricted model In each case, make note of the SSR Impos
27、ing the restrictions can be tricky will likely have to redefine variables again Example: Use same voting model as before Model is voteA = b0 + b1log(expendA) + b2log(expendB) + b3prtystrA + u now null is H0: b1 = 1, b3 = 0 Substituting in the restrictions: voteA = b0 + log(expendA) + b2log(expendB)
28、+ u, so Use voteA - log(expendA) = b0 + b2log(expendB) + u as restricted model F Statistic Summary Just as with t statistics, p-values can be calculated by looking up the percentile in the appropriate F distribution Stata will do this by entering: display fprob(q, n k 1, F), where the appropriate values of F, q,and n k 1 are used If only one exclusion is being tested, then F = t2, and the p-values will be the same
- 溫馨提示:
1: 本站所有資源如無特殊說明,都需要本地電腦安裝OFFICE2007和PDF閱讀器。圖紙軟件為CAD,CAXA,PROE,UG,SolidWorks等.壓縮文件請下載最新的WinRAR軟件解壓。
2: 本站的文檔不包含任何第三方提供的附件圖紙等,如果需要附件,請聯(lián)系上傳者。文件的所有權(quán)益歸上傳用戶所有。
3.本站RAR壓縮包中若帶圖紙,網(wǎng)頁內(nèi)容里面會有圖紙預(yù)覽,若沒有圖紙預(yù)覽就沒有圖紙。
4. 未經(jīng)權(quán)益所有人同意不得將文件中的內(nèi)容挪作商業(yè)或盈利用途。
5. 裝配圖網(wǎng)僅提供信息存儲空間,僅對用戶上傳內(nèi)容的表現(xiàn)方式做保護(hù)處理,對用戶上傳分享的文檔內(nèi)容本身不做任何修改或編輯,并不能對任何下載內(nèi)容負(fù)責(zé)。
6. 下載文件中如有侵權(quán)或不適當(dāng)內(nèi)容,請與我們聯(lián)系,我們立即糾正。
7. 本站不保證下載資源的準(zhǔn)確性、安全性和完整性, 同時也不承擔(dān)用戶因使用這些下載資源對自己和他人造成任何形式的傷害或損失。
最新文檔
- 2023年六年級數(shù)學(xué)下冊6整理和復(fù)習(xí)2圖形與幾何第7課時圖形的位置練習(xí)課件新人教版
- 2023年六年級數(shù)學(xué)下冊6整理和復(fù)習(xí)2圖形與幾何第1課時圖形的認(rèn)識與測量1平面圖形的認(rèn)識練習(xí)課件新人教版
- 2023年六年級數(shù)學(xué)下冊6整理和復(fù)習(xí)1數(shù)與代數(shù)第10課時比和比例2作業(yè)課件新人教版
- 2023年六年級數(shù)學(xué)下冊4比例1比例的意義和基本性質(zhì)第3課時解比例練習(xí)課件新人教版
- 2023年六年級數(shù)學(xué)下冊3圓柱與圓錐1圓柱第7課時圓柱的體積3作業(yè)課件新人教版
- 2023年六年級數(shù)學(xué)下冊3圓柱與圓錐1圓柱第1節(jié)圓柱的認(rèn)識作業(yè)課件新人教版
- 2023年六年級數(shù)學(xué)下冊2百分?jǐn)?shù)(二)第1節(jié)折扣和成數(shù)作業(yè)課件新人教版
- 2023年六年級數(shù)學(xué)下冊1負(fù)數(shù)第1課時負(fù)數(shù)的初步認(rèn)識作業(yè)課件新人教版
- 2023年六年級數(shù)學(xué)上冊期末復(fù)習(xí)考前模擬期末模擬訓(xùn)練二作業(yè)課件蘇教版
- 2023年六年級數(shù)學(xué)上冊期末豐收園作業(yè)課件蘇教版
- 2023年六年級數(shù)學(xué)上冊易錯清單十二課件新人教版
- 標(biāo)準(zhǔn)工時講義
- 2021年一年級語文上冊第六單元知識要點習(xí)題課件新人教版
- 2022春一年級語文下冊課文5識字測評習(xí)題課件新人教版
- 2023年六年級數(shù)學(xué)下冊6整理和復(fù)習(xí)4數(shù)學(xué)思考第1課時數(shù)學(xué)思考1練習(xí)課件新人教版