數(shù)字圖像處理,岡薩雷斯,課件英文版 Chapter05 圖像復(fù)原
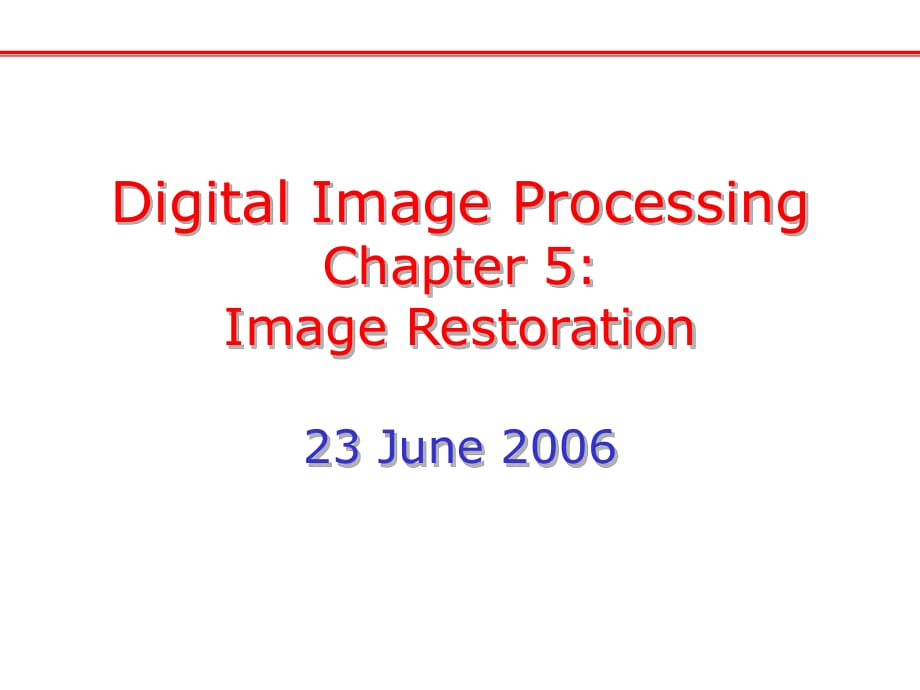


《數(shù)字圖像處理,岡薩雷斯,課件英文版 Chapter05 圖像復(fù)原》由會(huì)員分享,可在線閱讀,更多相關(guān)《數(shù)字圖像處理,岡薩雷斯,課件英文版 Chapter05 圖像復(fù)原(62頁(yè)珍藏版)》請(qǐng)?jiān)谘b配圖網(wǎng)上搜索。
1、Digital Image ProcessingChapter 5: Image Restoration23 June 2006 (Images from Rafael C. Gonzalez and Richard E. Wood, Digital Image Processing, 2nd Edition. Image restoration is to restore a degraded image back tothe original image while image enhancement is to manipulate the image so that it is sui
2、table for a specificapplication.Degradation model: ),(),(),(),( yxyxhyxfyxg where h(x,y) is a system that causes image distortion and(x,y) is noise. Noise cannot be predicted but can be approximately described instatistical way using the probability density function (PDF)Gaussian noise: 22 2/)(21)(
3、zezpRayleigh noise azfor 0 for )(2)( /)( 2 azeazbzp bazErlang (Gamma) noise 0zfor 0 0for )()!1()( 1 zeazb zazp azbb Exponential noiseUniform noiseImpulse (salt & pepper) noise azaezp )( otherwise 0 afor a-b1)( bzzp otherwise 0 for for )( bzP azPzp ba (Images from Rafael C. Gonzalez and Richard E. Wo
4、od, Digital Image Processing, 2nd Edition. PDF tells how mucheach z value occurs. (Images from Rafael C. Gonzalez and Richard E. Wood, Digital Image Processing, 2nd Edition. Original imageHistogram Degraded images),(),(),( yxyxfyxg (Images from Rafael C. Gonzalez and Richard E. Wood, Digital Image P
5、rocessing, 2nd Edition. Original imageHistogram Degraded images),(),(),( yxyxfyxg (Images from Rafael C. Gonzalez and Richard E. Wood, Digital Image Processing, 2nd Edition.Periodic noise looks like dotsIn the frequencydomain (Images from Rafael C. Gonzalez and Richard E. Wood, Digital Image Process
6、ing, 2nd Edition. We cannot use the image histogram to estimate noise PDF.It is better to use the histogram of one areaof an image that has constant intensity to estimate noise PDF. (Images from Rafael C. Gonzalez and Richard E. Wood, Digital Image Processing, 2nd Edition.Band reject filter Restored
7、 image Degraded image DFT Periodic noisecan be reduced bysetting frequencycomponentscorresponding to noise to zero. (Images from Rafael C. Gonzalez and Richard E. Wood, Digital Image Processing, 2nd Edition. Use to eliminate frequency components in some bandsPeriodic noise from theprevious slide tha
8、t is Filtered out. (Images from Rafael C. Gonzalez and Richard E. Wood, Digital Image Processing, 2nd Edition. A notch reject filter is used to eliminate some frequency components. (Images from Rafael C. Gonzalez and Richard E. Wood, Digital Image Processing, 2nd Edition. Degraded image DFT Notch fi
9、lter (freq. Domain) Restored imageNoise (Images from Rafael C. Gonzalez and Richard E. Wood, Digital Image Processing, 2nd Edition. Degraded image DFT(no shift) Restored imageNoiseDFT of noise Arithmetic mean filter or moving average filter (from Chapter 3) xySts tsgmnyxf ),( ),(1),(Geometric mean f
10、ilter mnSts xy tsgyxf 1),( ),(),( mn = size of moving window Degradation model: ),(),(),(),( yxyxhyxfyxg To remove this part (Images from Rafael C. Gonzalez and Richard E. Wood, Digital Image Processing, 2nd Edition. Original image Image corrupted by AWGNImage obtained using a 3x3geometric mean filt
11、erImage obtained using a 3x3arithmetic mean filterAWGN: Additive White Gaussian Noise Harmonic mean filter xySts tsgmnyxf ),( ),(1),(Contraharmonic mean filter xyxySts QSts Qtsg tsgyxf ),( ),( 1),( ),(),( mn = size of moving window Works well for salt noisebut fails for pepper noiseQ = the filter or
12、der Positive Q is suitable for eliminating pepper noise.Negative Q is suitable for eliminating salt noise.For Q = 0, the filter reduces to an arithmetic mean filter.For Q = -1, the filter reduces to a harmonic mean filter. (Images from Rafael C. Gonzalez and Richard E. Wood, Digital Image Processing
13、, 2nd Edition. Image corrupted by pepper noise with prob. = 0.1 Image corrupted by salt noise with prob. = 0.1Image obtained using a 3x3contra-harmonic mean filterWith Q = 1.5 Image obtained using a 3x3contra-harmonic mean filterWith Q=-1.5 (Images from Rafael C. Gonzalez and Richard E. Wood, Digita
14、l Image Processing, 2nd Edition. Image corrupted by pepper noise with prob. = 0.1 Image corrupted by salt noise with prob. = 0.1Image obtained using a 3x3contra-harmonic mean filterWith Q=-1.5 Image obtained using a 3x3contra-harmonic mean filterWith Q=1.5 subimageOriginal imageMoving window Statist
15、ic parametersMean, Median, Mode, Min, Max, Etc.Output image Median filter ),(median),( ),( tsgyxf xySts Max filter ),(max),( ),( tsgyxf xySts Min filter ),(min),( ),( tsgyxf xySts Midpoint filter ),(min),(max21),( ),(),( tsgtsgyxf xyxy StsSts Reduce “dark” noise (pepper noise)Reduce “bright” noise (
16、salt noise) A median filter is good for removing impulse, isolated noiseDegraded imageSalt noisePepper noise Movingwindow Sorted arraySalt noisePepper noise MedianFilter outputNormally, impulse noise has high magnitude and is isolated. When we sort pixels in the moving window, noise pixels are usual
17、ly at the ends of the array. Therefore, its rare that the noise pixel will be a median value. (Images from Rafael C. Gonzalez and Richard E. Wood, Digital Image Processing, 2nd Edition. Image corrupted by salt-and-pepper noise with pa=pb= 0.1 Images obtained using a 3x3 median filter1 423 (Images fr
18、om Rafael C. Gonzalez and Richard E. Wood, Digital Image Processing, 2nd Edition. Image corrupted by pepper noise with prob. = 0.1 Image corrupted by salt noise with prob. = 0.1Image obtained using a 3x3max filter Image obtained using a 3x3min filter xySts r tsgdmnyxf ),( ),(1),(where gr(s,t) repres
19、ent the remaining mn-d pixels after removing the d/2 highest and d/2 lowest values of g(s,t).This filter is useful in situations involving multiple typesof noise such as a combination of salt-and-pepper and Gaussian noise. Formula: (Images from Rafael C. Gonzalez and Richard E. Wood, Digital Image P
20、rocessing, 2nd Edition. Image corrupted by additiveuniform noiseImage obtained using a 5x5arithmetic mean filter Image additionallycorrupted by additivesalt-and-pepper noise1 22 Image obtained using a 5x5geometric mean filter2 Image corrupted by additiveuniform noiseImage obtained using a 5x5 median
21、 filter Image additionallycorrupted by additivesalt-and-pepper noise1 22 Image obtained using a 5x5alpha-trimmed mean filterwith d = 52 Image obtained using a 5x5arithmetic mean filter Image obtained using a 5x5geometric mean filterImage obtained using a 5x5 median filter Image obtained using a 5x5a
22、lpha-trimmed mean filterwith d = 5 -Filter behavior depends on statistical characteristics of local areas inside mxn moving window- More complex but superior performance compared with “fixed” filtersStatistical characteristics:General concept: xyStsL tsgmnm ),( ),(1Local mean:Local variance: xySts L
23、L mtsgmn ),( 22 ),(1 2Noise variance: Purpose: want to preserve edges1. If 2 is zero, No noisethe filter should return g(x,y) because g(x,y) = f(x,y)2. If L2 is high relative to 2, Edges (should be preserved), the filter should return the value close to g(x,y)3. If L2 = 2, Areas inside objectsthe fi
24、lter should return the arithmetic mean value m L LL myxgyxgyxf ),(),(),( 22Formula:Concept: (Images from Rafael C. Gonzalez and Richard E. Wood, Digital Image Processing, 2nd Edition. Image corrupted by additiveGaussian noise with zero meanand 2=1000 Imageobtained using a 7x7arithmeticmean filterIma
25、geobtained using a 7x7geometricmean filter Imageobtained using a 7x7adaptivenoise reduction filter Algorithm:Level A: A1= zmedian zminA2= zmedian zmaxIf A1 0 and A2 0, goto level BElse increase window sizeIf window size 0 and B2 0, return zxyElse return zmedianz min = minimum gray level value in Sxy
26、zmax = maximum gray level value in Sxyzmedian = median of gray levels in Sxyzxy = gray level value at pixel (x,y)Smax = maximum allowed size of Sxywhere Purpose: want to remove impulse noise while preserving edges Level A: A1= zmedian zmin A2= zmedian zmax Else Window is not big enough increase wind
27、ow sizeIf window size 0 and B2 0 and A2 0, goto level BLevel B: Determine whether zmedianis an impulse or notDetermine whether z xyis an impulse or not (Images from Rafael C. Gonzalez and Richard E. Wood, Digital Image Processing, 2nd Edition. Image corrupted by salt-and-pepper noise with pa=pb= 0.2
28、5 Image obtained using a 7x7median filter Image obtained using an adaptivemedian filter withSmax = 7More small details are preserved (Images from Rafael C. Gonzalez and Richard E. Wood, Digital Image Processing, 2nd Edition. Degradation model: ),(),(),(),( yxyxhyxfyxg Purpose: to estimate h(x,y) or
29、H(u,v) ),(),(),(),( vuNvuHvuFvuG Methods:1. Estimation by Image Observation2. Estimation by Experiment 3. Estimation by ModelingorWhy? If we know exactly h(x,y), regardless of noise, we can do deconvolution to get f(x,y) back from g(x,y). f(x,y) f(x,y)*h(x,y) g(x,y)SubimageReconstructedSubimage),( v
30、uGs ),( yxg s),( yxfsDFTDFT),( vuFs Restoration process byestimation Original image (unknown) Degraded image ),( ),(),(),( vuF vuGvuHvuH sss Estimated Transfer function ObservationThis case is used when weknow only g(x,y) and cannot repeat the experiment! Used when we have the same equipment set up
31、and can repeat the experiment.Input impulse image SystemH( ) Response image fromthe system ),( vuG ),( yxg),( yxA AyxADFT ),( A vuGvuH ),(),( DFTDFT (Images from Rafael C. Gonzalez and Richard E. Wood, Digital Image Processing, 2nd Edition. (Images from Rafael C. Gonzalez and Richard E. Wood, Digita
32、l Image Processing, 2nd Edition. Used when we know physical mechanism underlying the image formation process that can be expressed mathematically.AtmosphericTurbulence model 6/522 )(),( vukevuH Example:Original image Severe turbulencek = 0.00025k = 0.001 k = 0.0025Low turbulenceMild turbulence Assum
33、e that camera velocity is )(),( 00 tytxThe blurred image is obtained by dttyytxxfyxg T )(),(),( 000 where T = exposure time. dtdxdyetyytxxf dxdyedttyytxxf dxdyeyxgvuG T vyuxj vyuxjT vyuxj 0 )(200 )(20 00 )(2 )(),( )(),( ),(),( dtevuF dtevuF dtdxdyetyytxxfvuG T tvytuxjT tvytuxjT vyuxj 0 )()(20 )()(20
34、 )(200 00 00),( ),( )(),( ),( Then we get, the motion blurring transfer function:dtevuH T tvytuxj 0 )()(2 00),( For constant motion ),()(),( 00 btattytx )(0 )(2 )(sin()(),( vbuajT vbuaj evbuavbuaTdtevuH (Images from Rafael C. Gonzalez and Richard E. Wood, Digital Image Processing, 2nd Edition. For c
35、onstant motion )()(sin()(),( vbuajevbuavbuaTvuH Original image Motion blurred imagea = b = 0.1, T = 1 (Images from Rafael C. Gonzalez and Richard E. Wood, Digital Image Processing, 2nd Edition. after we obtain H(u,v), we can estimate F(u,v) by the inverse filter:),( ),(),(),( ),(),( vuH vuNvuFvuH vu
36、GvuF From degradation model: ),(),(),(),( vuNvuHvuFvuG Noise is enhancedwhen H(u,v) is small. To avoid the side effect of enhancing noise, we can apply this formulation to freq. component (u,v) with in a radius D 0 from the center of H(u,v).In practical, the inverse filter is notPopularly used. 6/52
37、2 )(0025.0),( vuevuH Original imageBlurred imageDue to Turbulence Result of applyingthe full filterResult of applyingthe filter with D0=70 Result of applyingthe filter with D0=40 Result of applyingthe filter with D0=85 (Images from Rafael C. Gonzalez and Richard E. Wood, Digital Image Processing, 2n
38、d Edition. Objective: optimize mean square error: 22 )( ffEe ),(),(/),(),( ),(),(1 ),(),(/),(),( ),( ),(),(),(),( ),(),(),( 2 22 * 2 * vuGvuSvuSvuH vuHvuH vuGvuSvuSvuH vuH vuGvuSvuHvuS vuSvuHvuF fff f Wiener Filter Formula:whereH(u,v) = Degradation functionS (u,v) = Power spectrum of noiseSf(u,v) =
39、Power spectrum of the undegraded image ),(),(/),(),( ),(),(1),( 2 2 vuGvuSvuSvuH vuHvuHvuF f Wiener Filter Formula:Approximated Formula: ),(),( ),(),(1),( 2 2 vuGKvuH vuHvuHvuF Difficult to estimatePractically, K is chosen manually to obtained the best visual result! Original imageBlurred imageDue t
40、o Turbulence Result of the full inverse filter Result of the inverse filter with D0=70 Result of the full Wiener filter Original image Result of the inverse filter with D0=70 Result of the Wiener filterBlurred imageDue to Turbulence (Images from Rafael C. Gonzalez and Richard E. Wood, Digital Image
41、Processing, 2nd Edition. Image degradedby motion blur +AWGN Result of theinverse filter Result of theWiener filter2=650 2=3252=130 Note: K is chosenmanually Degradation model: ),(),(),(),( yxyxhyxfyxg Written in a matrix formH fg Objective: to find the minimum of a criterion function 10 10 22 ),(Mx
42、Ny yxfCSubject to the constraint 22 fHg ),(),(),( ),(),( 22* vuGvuPvuH vuHvuF We get a constrained least square filterwhere P(u,v) = Fourier transform of p(x,y) = 010 141 010where www T2 ),(),(),( ),(),( 22* vuGvuPvuH vuHvuF Constrained least square filter is adaptively adjusted to achieve the best
43、result.Results from the previous slide obtained from the constrained least square filter Image degradedby motion blur +AWGN Result of theConstrainedLeast square filter Result of theWiener filter2=650 2=3252=130 Define fHgr It can be shown that 2)( rrr TWe want to adjust gamma so that a 22 r where a
44、= accuracy factor1. Specify an initial value of 2. Compute3. Stop if is satisfiedOtherwise return step 2 after increasing if or decreasing ifUse the new value of to recompute 11 2r a 22 r a 22 r ),(),(),( ),(),( 22* vuGvuPvuH vuHvuF ),(),(),( ),(),( 22* vuGvuPvuH vuHvuF ),(),(),(),( vuFvuHvuGvuR 10
45、10 22 ),(1 Mx Ny yxrMNr 10 10 22 ),(1 Mx Ny myxMN 10 10 ),(1 Mx Ny yxMNm mMN 22 2 2rFor computingFor computing (Images from Rafael C. Gonzalez and Richard E. Wood, Digital Image Processing, 2nd Edition. Original imageBlurred imageDue to Turbulence Results obtained from constrained least square filte
46、rsUse wrong noise parameters Correct parameters:Initial = 10-5Correction factor = 10-6a = 0.252 = 10-5Wrong noise parameter2 = 10-2Use correct noise parameters ),(),( ),(),( ),(),( ),(),( 12 *2* vuGvuS vuSvuH vuHvuH vuHvuF f This filter represents a family of filters combined into a single expressio
47、n = 1 the inverse filter = 0 the Parametric Wiener filter = 0, = 1 the standard Wiener filter = 1, 0.5 More like the Wiener filterAnother name: the spectrum equalization filter These transformations are often called rubber-sheet transformations: Printing an image on a rubber sheet and then stretch t
48、his sheet accordingto some predefine set of rules. A geometric transformation consists of 2 basic operations:1. A spatial transformation : Define how pixels are to be rearranged in the spatiallytransformed image.2. G ray level interpolation :Assign gray level values to pixels in the spatiallytransfo
49、rmed image. Distorted image g1. Select coordinate (x,y) in f to be restored2. Compute ),( yxrx ),( yxsy 3. Go to pixel in a distorted image g),( yx Image f to be restored 4. get pixel value atBy gray level interpolation ),( yxg 5. store that value in pixel f(x,y)1 3 5),( yx ),( yx (Images from Rafae
50、l C. Gonzalez and Richard E. Wood, Digital Image Processing, 2nd Edition. To map between pixel coordinate (x,y) of f and pixel coordinate (x,y) of g ),( yxrx ),( yxsy For a bilinear transformation mapping between a pair of Quadrilateral regions 4321),( cxycycxcyxrx 8765),( cxycycxcyxsy ),( yx ),( yx
51、To obtain r(x,y) and s(x,y), we need to know 4 pairs of coordinates and its correspondingwhich are called tiepoints. ),( yx ),( yx (Images from Rafael C. Gonzalez and Richard E. Wood, Digital Image Processing, 2nd Edition. Since may not be at an integer coordinate, we need to Interpolate the value o
52、f ),( yx ),( yxg Example interpolation methods that can be used:1. Nearest neighbor selection2. Bilinear interpolation3. Bicubic interpolation Original image and tiepoints Tiepoints of distortedimageDistorted image Restored image Use nearestneighbor intepolation (Images from Rafael C. Gonzalez and R
53、ichard E. Wood, Digital Image Processing, 2nd Edition. Original image and tiepoints Tiepoints of distortedimageDistorted image Restored image Use bilinear intepolation (Images from Rafael C. Gonzalez and Richard E. Wood, Digital Image Processing, 2nd Edition. (Images from Rafael C. Gonzalez and Richard E. Wood, Digital Image Processing, 2nd Edition. Original image Geometrically distortedimageDifference between 2 above images Restored image Use the sameSpatial Trans.as in the previousexample
- 溫馨提示:
1: 本站所有資源如無(wú)特殊說(shuō)明,都需要本地電腦安裝OFFICE2007和PDF閱讀器。圖紙軟件為CAD,CAXA,PROE,UG,SolidWorks等.壓縮文件請(qǐng)下載最新的WinRAR軟件解壓。
2: 本站的文檔不包含任何第三方提供的附件圖紙等,如果需要附件,請(qǐng)聯(lián)系上傳者。文件的所有權(quán)益歸上傳用戶所有。
3.本站RAR壓縮包中若帶圖紙,網(wǎng)頁(yè)內(nèi)容里面會(huì)有圖紙預(yù)覽,若沒(méi)有圖紙預(yù)覽就沒(méi)有圖紙。
4. 未經(jīng)權(quán)益所有人同意不得將文件中的內(nèi)容挪作商業(yè)或盈利用途。
5. 裝配圖網(wǎng)僅提供信息存儲(chǔ)空間,僅對(duì)用戶上傳內(nèi)容的表現(xiàn)方式做保護(hù)處理,對(duì)用戶上傳分享的文檔內(nèi)容本身不做任何修改或編輯,并不能對(duì)任何下載內(nèi)容負(fù)責(zé)。
6. 下載文件中如有侵權(quán)或不適當(dāng)內(nèi)容,請(qǐng)與我們聯(lián)系,我們立即糾正。
7. 本站不保證下載資源的準(zhǔn)確性、安全性和完整性, 同時(shí)也不承擔(dān)用戶因使用這些下載資源對(duì)自己和他人造成任何形式的傷害或損失。
最新文檔
- 川渝旅游日記成都重慶城市介紹推薦景點(diǎn)美食推薦
- XX國(guó)有企業(yè)黨委書記個(gè)人述責(zé)述廉報(bào)告及2025年重點(diǎn)工作計(jì)劃
- 世界濕地日濕地的含義及價(jià)值
- 20XX年春節(jié)節(jié)后復(fù)工安全生產(chǎn)培訓(xùn)人到場(chǎng)心到崗
- 大唐女子圖鑒唐朝服飾之美器物之美繪畫之美生活之美
- 節(jié)后開(kāi)工第一課輕松掌握各要點(diǎn)節(jié)后常見(jiàn)的八大危險(xiǎn)
- 廈門城市旅游介紹廈門景點(diǎn)介紹廈門美食展示
- 節(jié)后開(kāi)工第一課復(fù)工復(fù)產(chǎn)十注意節(jié)后復(fù)工十檢查
- 傳統(tǒng)文化百善孝為先孝道培訓(xùn)
- 深圳城市旅游介紹景點(diǎn)推薦美食探索
- 節(jié)后復(fù)工安全生產(chǎn)培訓(xùn)勿忘安全本心人人講安全個(gè)個(gè)會(huì)應(yīng)急
- 預(yù)防性維修管理
- 常見(jiàn)閥門類型及特點(diǎn)
- 設(shè)備預(yù)防性維修
- 2.乳化液泵工理論考試試題含答案